Data-driven modeling and learning in science and engineering
Author(s)
Montáns, Francisco J.; Chinesta, Francisco; Gomez-Bombarelli, Rafael; Kutz, J. Nathan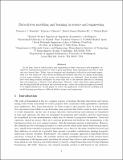
DownloadAccepted version (731.9Kb)
Publisher with Creative Commons License
Publisher with Creative Commons License
Creative Commons Attribution
Terms of use
Metadata
Show full item recordAbstract
In the past, data in which science and engineering is based, was scarce and frequently obtained by experiments proposed to verify a given hypothesis. Each experiment was able to yield only very limited data. Today, data is abundant and abundantly collected in each single experiment at a very small cost. Data-driven modeling and scientific discovery is a change of paradigm on how many problems, both in science and engineering, are addressed. Some scientific fields have been using artificial intelligence for some time due to the inherent difficulty in obtaining laws and equations to describe some phenomena. However, today data-driven approaches are also flooding fields like mechanics and materials science, where the traditional approach seemed to be highly satisfactory. In this paper we review the application of data-driven modeling and model learning procedures to different fields in science and engineering.
Date issued
2019-11Department
Massachusetts Institute of Technology. Department of Materials Science and EngineeringJournal
Comptes Rendus Mécanique
Publisher
Elsevier BV
Citation
Montáns, Francisco J. et al. "Data-driven modeling and learning in science and engineering." Comptes Rendus Mécanique 347, 11 (November 2019): 845-855 © 2019 Académie des sciences
Version: Author's final manuscript
ISSN
1631-0721