Patient-Specific Conditional Joint Models of Shape, Image Features and Clinical Indicators
Author(s)
Egger, Bernhard; Schirmer, Markus; Dubost, Florian; Nardin, Marco J.; Rost, Natalia S.; Golland, Polina; ... Show more Show less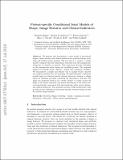
DownloadSubmitted version (2.616Mb)
Open Access Policy
Open Access Policy
Creative Commons Attribution-Noncommercial-Share Alike
Terms of use
Metadata
Show full item recordAbstract
We propose and demonstrate a joint model of anatomical shapes, image features and clinical indicators for statistical shape modeling and medical image analysis. The key idea is to employ a copula model to separate the joint dependency structure from the marginal distributions of variables of interest. This separation provides flexibility on the assumptions made during the modeling process. The proposed method can handle binary, discrete, ordinal and continuous variables. We demonstrate a simple and efficient way to include binary, discrete and ordinal variables into the modeling. We build Bayesian conditional models based on observed partial clinical indicators, features or shape based on Gaussian processes capturing the dependency structure. We apply the proposed method on a stroke dataset to jointly model the shape of the lateral ventricles, the spatial distribution of the white matter hyperintensity associated with periventricular white matter disease, and clinical indicators. The proposed method yields interpretable joint models for data exploration and patient-specific statistical shape models for medical image analysis.
Description
Part of the Lecture Notes in Computer Science book series (LNCS, volume 11767).
Date issued
2019-10Department
Massachusetts Institute of Technology. Computer Science and Artificial Intelligence Laboratory; Massachusetts Institute of Technology. Department of Electrical Engineering and Computer ScienceJournal
Lecture Notes in Computer Science
Publisher
Springer International Publishing
Citation
Egger, Bernhard et al. "Patient-Specific Conditional Joint Models of Shape, Image Features and Clinical Indicators." International Conference on Medical Image Computing and Computer-Assisted Intervention, Lecture Notes in Computer Science, 11767, Springer Nature, 2019, 93-101. © 2019 Springer Nature
Version: Original manuscript
ISBN
9783030322502
9783030322519
ISSN
0302-9743
1611-3349