Implicit posterior variational inference for deep Gaussian processes
Author(s)
Jaillet, Patrick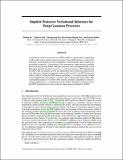
DownloadPublished version (6.830Mb)
Publisher Policy
Publisher Policy
Article is made available in accordance with the publisher's policy and may be subject to US copyright law. Please refer to the publisher's site for terms of use.
Terms of use
Metadata
Show full item recordAbstract
A multi-layer deep Gaussian process (DGP) model is a hierarchical composition of GP models with a greater expressive power. Exact DGP inference is intractable, which has motivated the recent development of deterministic and stochastic approximation methods. Unfortunately, the deterministic approximation methods yield a biased posterior belief while the stochastic one is computationally costly. This paper presents an implicit posterior variational inference (IPVI) framework for DGPs that can ideally recover an unbiased posterior belief and still preserve time efficiency. Inspired by generative adversarial networks, our IPVI framework achieves this by casting the DGP inference problem as a two-player game in which a Nash equilibrium, interestingly, coincides with an unbiased posterior belief. This consequently inspires us to devise a best-response dynamics algorithm to search for a Nash equilibrium (i.e., an unbiased posterior belief). Empirical evaluation shows that IPVI outperforms the state-of-the-art approximation methods for DGPs.
Date issued
2019-12Department
Massachusetts Institute of Technology. Department of Electrical Engineering and Computer ScienceJournal
Advances in Neural Information Processing Systems
Publisher
Morgan Kaufmann Publishers
Citation
Yu, , Haibin et al. “Implicit posterior variational inference for deep Gaussian processes.” Advances in Neural Information Processing Systems, 32 (December 2019) © 2019 The Author(s)
Version: Final published version
ISSN
1049-5258