DeepLigand: accurate prediction of MHC class I ligands using peptide embedding
Author(s)
Zeng, Haoyang; Gifford, David K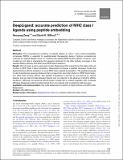
DownloadPublished version (691.9Kb)
Publisher with Creative Commons License
Publisher with Creative Commons License
Creative Commons Attribution
Terms of use
Metadata
Show full item recordAbstract
Motivation: The computational modeling of peptide display by class I major histocompatibility complexes (MHCs) is essential for peptide-based therapeutics design. Existing computational methods for peptide-display focus on modeling the peptide-MHC-binding affinity. However, such models are not able to characterize the sequence features for the other cellular processes in the peptide display pathway that determines MHC ligand selection. Results: We introduce a semi-supervised model, DeepLigand that outperforms the state-of-the-art models in MHC Class I ligand prediction. DeepLigand combines a peptide language model and peptide binding affinity prediction to score MHC class I peptide presentation. The peptide language model characterizes sequence features that correspond to secondary factors in MHC ligand selection other than binding affinity. The peptide embedding is learned by pre-training on natural ligands, and can discriminate between ligands and non-ligands in the absence of binding affinity prediction. Although conventional affinity-based models fail to classify peptides with moderate affinities, DeepLigand discriminates ligands from non-ligands with consistently high accuracy.
Date issued
2019-07Department
Massachusetts Institute of Technology. Department of Electrical Engineering and Computer ScienceJournal
Bioinformatics
Publisher
Oxford University Press (OUP)
Citation
Zeng, Haoyang and David K. Gifford. “DeepLigand: accurate prediction of MHC class I ligands using peptide embedding.” Bioinformatics, 35, 14 (July 2019): i278–i283 © 2019 The Author(s)
Version: Final published version
ISSN
1367-4803