A Unified Evaluation of Two-Candidate Ballot-Polling Election Auditing Methods
Author(s)
Huang, Zhuoqun; Rivest, Ronald L; Stark, Philip B.; Teague, Vanessa J.; Vukcevic, Damjan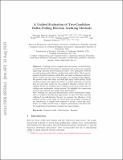
DownloadSubmitted version (449.3Kb)
Open Access Policy
Open Access Policy
Creative Commons Attribution-Noncommercial-Share Alike
Terms of use
Metadata
Show full item recordAbstract
Counting votes is complex and error-prone. Several statistical methods have been developed to assess election accuracy by manually inspecting randomly selected physical ballots. Two ‘principled’ methods are risk-limiting audits (RLAs) and Bayesian audits (BAs). RLAs use frequentist statistical inference while BAs are based on Bayesian inference. Until recently, the two have been thought of as fundamentally different. We present results that unify and shed light upon ‘ballot-polling’ RLAs and BAs (which only require the ability to sample uniformly at random from all cast ballot cards) for two-candidate plurality contests, that are building blocks for auditing more complex social choice functions, including some preferential voting systems. We highlight the connections between the methods and explore their performance. First, building on a previous demonstration of the mathematical equivalence of classical and Bayesian approaches, we show that BAs, suitably calibrated, are risk-limiting. Second, we compare the efficiency of the methods across a wide range of contest sizes and margins, focusing on the distribution of sample sizes required to attain a given risk limit. Third, we outline several ways to improve performance and show how the mathematical equivalence explains the improvements.
Date issued
2020-09Department
Massachusetts Institute of Technology. Computer Science and Artificial Intelligence LaboratoryJournal
Lecture Notes in Computer Science
Publisher
Springer International Publishing
Citation
Huang, Zhuoqun et al. "A Unified Evaluation of Two-Candidate Ballot-Polling Election Auditing Methods."
E-Vote-ID: International Joint Conference on Electronic Voting, Lecture Notes in Computer Science, 12455, Springer International Publishing, 2020, 112-128. © 2020 Springer Nature
Version: Original manuscript
ISBN
9783030603465
9783030603472
ISSN
0302-9743
1611-3349