Robustness may be at odds with accuracy
Author(s)
Tsipras, Dimitris; Santurkar, Shibani (Shibani Vinay); Engstrom, Logan G.; Turner, Alexander M.; Madry, Aleksander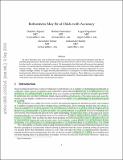
DownloadAccepted version (5.904Mb)
Open Access Policy
Open Access Policy
Creative Commons Attribution-Noncommercial-Share Alike
Terms of use
Metadata
Show full item recordAbstract
We show that there exists an inherent tension between the goal of adversarial robustness and that of standard generalization. Specifically, training robust models may not only be more resource-consuming, but also lead to a reduction of standard accuracy. We demonstrate that this trade-off between the standard accuracy of a model and its robustness to adversarial perturbations provably exists even in a fairly simple and natural setting. These findings also corroborate a similar phenomenon observed in practice. Further, we argue that this phenomenon is a consequence of robust classifiers learning fundamentally different feature representations than standard classifiers. These differences, in particular, seem to result in unexpected benefits: the features learned by robust models tend to align better with salient data characteristics and human perception.
Date issued
2019-04Department
Massachusetts Institute of Technology. Department of Electrical Engineering and Computer ScienceJournal
7th International Conference on Learning Representations, ICLR 2019
Publisher
ICLR
Citation
Tsipras, Dimitris et al. “Robustness may be at odds with accuracy.” Paper presented at 7th International Conference on Learning Representations, ICLR 2019, New Orleans, Louisiana, May 6 - 9, 2019, ICLR © 2019 The Author(s)
Version: Author's final manuscript