Music Gesture for Visual Sound Separation
Author(s)
Gan, Chuang; Huang, Deng; Zhao, Hang; Tenenbaum, Joshua B; Torralba, Antonio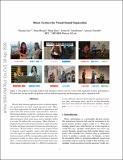
DownloadSubmitted version (10.10Mb)
Open Access Policy
Open Access Policy
Creative Commons Attribution-Noncommercial-Share Alike
Terms of use
Metadata
Show full item recordAbstract
Recent deep learning approaches have achieved impressive performance on visual sound separation tasks. However, these approaches are mostly built on appearance and optical flow like motion feature representations, which exhibit limited abilities to find the correlations between audio signals and visual points, especially when separating multiple instruments of the same types, such as multiple violins in a scene. To address this, we propose ''Music Gesture,' a keypoint-based structured representation to explicitly model the body and finger movements of musicians when they perform music. We first adopt a context-aware graph network to integrate visual semantic context with body dynamics and then apply an audio-visual fusion model to associate body movements with the corresponding audio signals. Experimental results on three music performance datasets show: 1) strong improvements upon benchmark metrics for hetero-musical separation tasks (i.e. different instruments); 2) new ability for effective homo-musical separation for piano, flute, and trumpet duets, which to our best knowledge has never been achieved with alternative methods.
Date issued
2020-08Department
MIT-IBM Watson AI Lab; Massachusetts Institute of Technology. Department of Electrical Engineering and Computer ScienceJournal
2020 IEEE/CVF Conference on Computer Vision and Pattern Recognition
Publisher
Institute of Electrical and Electronics Engineers (IEEE)
Citation
Gan, Chuang et al. "Music Gesture for Visual Sound Separation." 2020 IEEE/CVF Conference on Computer Vision and Pattern Recognition, June 2020, Seattle, Washingston, Institute of Electrical and Electronics Engineers, August 2020. © 2020 IEEE
Version: Original manuscript
ISBN
9781728171685