Maximum Likelihood Embedding of Logistic Random Dot Product Graphs
Author(s)
M´edard, Muriel; Feizi, Soheil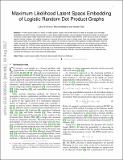
DownloadSubmitted version (1.272Mb)
Open Access Policy
Open Access Policy
Creative Commons Attribution-Noncommercial-Share Alike
Terms of use
Metadata
Show full item recordAbstract
A latent space model for a family of random graphs assigns real-valued vectors to nodes of the graph such that edge probabilities are determined by latent positions. Latent space models provide a natural statistical framework for graph visualizing and clustering. A latent space model of particular interest is the Random Dot Product Graph (RDPG), which can be fit using an efficient spectral method; however, this method is based on a heuristic that can fail, even in simple cases. Here, we consider a closely related latent space model, the Logistic RDPG, which uses a logistic link function to map from latent positions to edge likelihoods. Over this model, we show that asymptotically exact maximum likelihood inference of latent position vectors can be achieved using an efficient spectral method. Our method involves computing top eigenvectors of a normalized adjacency matrix and scaling eigenvectors using a regression step. The novel regression scaling step is an essential part of the proposed method. In simulations, we show that our proposed method is more accurate and more robust than common practices. We also show the effectiveness of our approach over standard real networks of the karate club and political blogs.
Date issued
2020-04Department
Massachusetts Institute of Technology. Department of Electrical Engineering and Computer ScienceJournal
Proceedings of the AAAI Conference on Artificial Intelligence
Publisher
Association for the Advancement of Artificial Intelligence (AAAI)
Citation
O’Connor, Luke et al. “Maximum Likelihood Embedding of Logistic Random Dot Product Graphs.” Proceedings of the AAAI Conference on Artificial Intelligence, 34, 4 (April 2020): 5289-5297 © 2020 The Author(s)
Version: Original manuscript
ISSN
2159-5399