An information-theoretic approach to unsupervised feature selection for high-dimensional data
Author(s)
Huang, Shao-Lun; Zhang, Lin; Zheng, Lizhong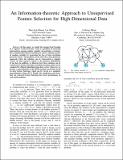
DownloadAccepted version (354.2Kb)
Open Access Policy
Open Access Policy
Creative Commons Attribution-Noncommercial-Share Alike
Terms of use
Metadata
Show full item recordAbstract
In this paper, we model the unsupervised learning of a sequence of observed data vector as a problem of extracting joint patterns among random variables. In particular, we formulate an information-theoretic problem to extract common features of random variables by measuring the loss of total correlation given the feature. This problem can be solved by a local geometric approach, where the solutions can be represented as singular vectors of some matrices related to the pairwise distributions of the data. In addition, we illustrate how these solutions can be transferred to feature functions in machine learning, which can be computed by efficient algorithms from data vectors. Moreover, we present a generalization of the HGR maximal correlation based on these feature functions, which can be viewed as a nonlinear generalization to linear PCA. Finally, the simulation result shows that our extracted feature functions have great performance in real-world problems.
Date issued
2018-02Department
Massachusetts Institute of Technology. Department of Electrical Engineering and Computer ScienceJournal
2017 IEEE Information Theory Workshop (ITW)
Publisher
Institute of Electrical and Electronics Engineers (IEEE)
Citation
Huang, Shao-Lun et al. "An information-theoretic approach to unsupervised feature selection for high-dimensional data." 2017 IEEE Information Theory Workshop, November 2017, Kaohsiung, Taiwan, Institute of Electrical and Electronics Engineers, February 2018. © 2017 IEEE
Version: Author's final manuscript
ISBN
9781509030972