Early Detection of Red Palm Weevil, Rhynchophorus ferrugineus (Olivier), Infestation Using Data Mining
Author(s)
Kurdi, Heba; Al-Aldawsari, Amal; Al-Turaiki, Isra; Aldawood, Abdulrahman S.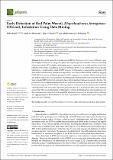
Downloadplants-10-00095-v2.pdf (485.1Kb)
Publisher with Creative Commons License
Publisher with Creative Commons License
Creative Commons Attribution
Terms of use
Metadata
Show full item recordAbstract
In the past 30 years, the red palm weevil (RPW), <i>Rhynchophorus ferrugineus</i> (Olivier), a pest that is highly destructive to all types of palms, has rapidly spread worldwide. However, detecting infestation with the RPW is highly challenging because symptoms are not visible until the death of the palm tree is inevitable. In addition, the use of automated RPW weevil identification tools to predict infestation is complicated by a lack of RPW datasets. In this study, we assessed the capability of 10 state-of-the-art data mining classification algorithms, Naive Bayes (NB), KSTAR, AdaBoost, bagging, PART, J48 Decision tree, multilayer perceptron (MLP), support vector machine (SVM), random forest, and logistic regression, to use plant-size and temperature measurements collected from individual trees to predict RPW infestation in its early stages before significant damage is caused to the tree. The performance of the classification algorithms was evaluated in terms of accuracy, precision, recall, and F-measure using a real RPW dataset. The experimental results showed that infestations with RPW can be predicted with an accuracy up to 93%, precision above 87%, recall equals 100%, and F-measure greater than 93% using data mining. Additionally, we found that temperature and circumference are the most important features for predicting RPW infestation. However, we strongly call for collecting and aggregating more RPW datasets to run more experiments to validate these results and provide more conclusive findings.
Date issued
2021-01-06Department
Massachusetts Institute of Technology. Department of Mechanical EngineeringPublisher
Multidisciplinary Digital Publishing Institute
Citation
Plants 10 (1): 95 (2021)
Version: Final published version