Space Objects Classification via Light-Curve Measurements Using Deep Convolutional Neural Networks
Author(s)
Linares, Richard; Furfaro, Roberto; Reddy, Vishnu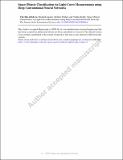
Download40295_2019_208_ReferencePDF.pdf (3.923Mb)
Open Access Policy
Open Access Policy
Creative Commons Attribution-Noncommercial-Share Alike
Terms of use
Metadata
Show full item recordAbstract
Abstract
This work presents a data-driven method for the classification of light curve measurements of Space Objects (SOs) based on a deep learning approach. Here, we design, train, and validate a Convolutional Neural Network (CNN) capable of learning to classify SOs from collected light-curve measurements. The proposed methodology relies on a physics-based model capable of accurately representing SO reflected light as a function of time, size, shape, and state of motion. The model generates thousands of light-curves per selected class of SO, which are employed to train a deep CNN to learn the functional relationship. between light-curves and SO classes. Additionally, a deep CNN is trained using real SO light-curves to evaluate the performance on real data, but limited training set. The CNNs are compared with more conventional machine learning techniques (bagged trees, support vector machines) and are shown to outperform such methods, especially when trained on real data.
Date issued
2020-03-12Department
Massachusetts Institute of Technology. Department of Aeronautics and AstronauticsPublisher
Springer US