Pareto gamuts
Author(s)
Makatura, Liane; Guo, Minghao; Schulz, Adriana; Solomon, Justin; Matusik, Wojciech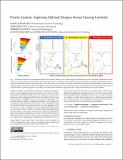
DownloadPublished version (34.20Mb)
Publisher with Creative Commons License
Publisher with Creative Commons License
Creative Commons Attribution
Terms of use
Metadata
Show full item recordAbstract
<jats:p>
Manufactured parts are meticulously engineered to perform well with respect to several conflicting metrics, like weight, stress, and cost. The best achievable trade-offs reside on the
<jats:italic>Pareto front</jats:italic>
, which can be discovered via performance-driven optimization. The objectives that define this Pareto front often incorporate assumptions about the
<jats:italic>context</jats:italic>
in which a part will be used, including loading conditions, environmental influences, material properties, or regions that must be preserved to interface with a surrounding assembly. Existing multi-objective optimization tools are only equipped to study one context at a time, so engineers must run independent optimizations for each context of interest. However, engineered parts frequently appear in many contexts: wind turbines must perform well in many wind speeds, and a bracket might be optimized several times with its bolt-holes fixed in different locations on each run. In this paper, we formulate a framework for variable-context multi-objective optimization. We introduce the
<jats:italic>Pareto gamut</jats:italic>
, which captures Pareto fronts over a range of contexts. We develop a global/local optimization algorithm to discover the Pareto gamut directly, rather than discovering a single fixed-context "slice" at a time. To validate our method, we adapt existing multi-objective optimization benchmarks to contextual scenarios. We also demonstrate the practical utility of Pareto gamut exploration for several engineering design problems.
</jats:p>
Date issued
2021-08Department
Massachusetts Institute of Technology. Department of Electrical Engineering and Computer Science; Massachusetts Institute of Technology. Computer Science and Artificial Intelligence Laboratory; Massachusetts Institute of Technology. Center for Computational Science and EngineeringJournal
ACM Transactions on Graphics
Publisher
Association for Computing Machinery (ACM)