Analyzing Learned Molecular Representations for Property Prediction
Author(s)
Yang, Kevin; Swanson, Kyle; Jin, Wengong; Coley, Connor; Eiden, Philipp; Gao, Hua; Guzman-Perez, Angel; Hopper, Timothy; Kelley, Brian; Mathea, Miriam; Palmer, Andrew; Settels, Volker; Jaakkola, Tommi; Jensen, Klavs; Barzilay, Regina; ... Show more Show less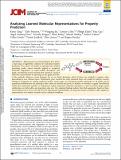
DownloadAccepted version (2.976Mb)
Publisher Policy
Publisher Policy
Article is made available in accordance with the publisher's policy and may be subject to US copyright law. Please refer to the publisher's site for terms of use.
Terms of use
Metadata
Show full item recordAbstract
© 2019 American Chemical Society. Advancements in neural machinery have led to a wide range of algorithmic solutions for molecular property prediction. Two classes of models in particular have yielded promising results: neural networks applied to computed molecular fingerprints or expert-crafted descriptors and graph convolutional neural networks that construct a learned molecular representation by operating on the graph structure of the molecule. However, recent literature has yet to clearly determine which of these two methods is superior when generalizing to new chemical space. Furthermore, prior research has rarely examined these new models in industry research settings in comparison to existing employed models. In this paper, we benchmark models extensively on 19 public and 16 proprietary industrial data sets spanning a wide variety of chemical end points. In addition, we introduce a graph convolutional model that consistently matches or outperforms models using fixed molecular descriptors as well as previous graph neural architectures on both public and proprietary data sets. Our empirical findings indicate that while approaches based on these representations have yet to reach the level of experimental reproducibility, our proposed model nevertheless offers significant improvements over models currently used in industrial workflows.
Date issued
2019Department
Massachusetts Institute of Technology. Computer Science and Artificial Intelligence LaboratoryJournal
Journal of Chemical Information and Modeling
Publisher
American Chemical Society (ACS)