Graduated Non-Convexity for Robust Spatial Perception: From Non-Minimal Solvers to Global Outlier Rejection
Author(s)
Yang, Heng; Antonante, Pasquale; Tzoumas, Vasileios; Carlone, Luca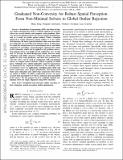
DownloadAccepted version (3.661Mb)
Open Access Policy
Open Access Policy
Creative Commons Attribution-Noncommercial-Share Alike
Terms of use
Metadata
Show full item recordAbstract
© 2016 IEEE. Semidefinite Programming (SDP) and Sums-of-Squ-ares (SOS) relaxations have led to certifiably optimal non-minimal solvers for several robotics and computer vision problems. However, most non-minimal solvers rely on least squares formulations, and, as a result, are brittle against outliers. While a standard approach to regain robustness against outliers is to use robust cost functions, the latter typically introduce other non-convexities, preventing the use of existing non-minimal solvers. In this letter, we enable the simultaneous use of non-minimal solvers and robust estimation by providing a general-purpose approach for robust global estimation, which can be applied to any problem where a non-minimal solver is available for the outlier-free case. To this end, we leverage the Black-Rangarajan duality between robust estimation and outlier processes (which has been traditionally applied to early vision problems), and show that graduated non-convexity (GNC) can be used in conjunction with non-minimal solvers to compute robust solutions, without requiring an initial guess. we demonstrate the resulting robust non-minimal solvers in applications, including point cloud and mesh registration, pose graph optimization, and image-based object pose estimation (also called shape alignment). Our solvers are robust to 70-80% of outliers, outperform RANSAC, are more accurate than specialized local solvers, and faster than specialized global solvers. We also propose the first certifiably optimal non-minimal solver for shape alignment using SOS relaxation.
Date issued
2020Department
Massachusetts Institute of Technology. Laboratory for Information and Decision SystemsJournal
IEEE Robotics and Automation Letters
Publisher
Institute of Electrical and Electronics Engineers (IEEE)