Privacy with Estimation Guarantees
Author(s)
Wang, Hao; Vo, Lisa; Calmon, Flavio P; Medard, Muriel; Duffy, Ken R; Varia, Mayank; ... Show more Show less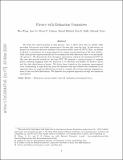
DownloadAccepted version (1.004Mb)
Open Access Policy
Open Access Policy
Creative Commons Attribution-Noncommercial-Share Alike
Terms of use
Metadata
Show full item recordAbstract
© 1963-2012 IEEE. We study the central problem in data privacy: how to share data with an analyst while providing both privacy and utility guarantees to the user that owns the data. In this setting, we present an estimation-theoretic analysis of the privacy-utility trade-off (PUT). Here, an analyst is allowed to reconstruct (in a mean-squared error sense) certain functions of the data (utility), while other private functions should not be reconstructed with distortion below a certain threshold (privacy). We demonstrate how chi-square information captures the fundamental PUT in this case and provide bounds for the best PUT. We propose a convex program to compute privacy-assuring mappings when the functions to be disclosed and hidden are known a priori and the data distribution is known. We derive lower bounds on the minimum mean-squared error of estimating a target function from the disclosed data and evaluate the robustness of our approach when an empirical distribution is used to compute the privacy-assuring mappings instead of the true data distribution. We illustrate the proposed approach through two numerical experiments.
Date issued
2019Department
Massachusetts Institute of Technology. Department of Electrical Engineering and Computer ScienceJournal
IEEE Transactions on Information Theory
Publisher
Institute of Electrical and Electronics Engineers (IEEE)