Convolution neural network model with improved pooling strategy and feature selection for weld defect recognition
Author(s)
Jiang, Hongquan; Hu, Qihang; Zhi, Zelin; Gao, Jianmin; Gao, Zhiyong; Wang, Rongxi; He, Shuai; Li, Hua; ... Show more Show less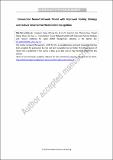
Download40194_2020_1027_ReferencePDF.pdf (2.498Mb)
Publisher Policy
Publisher Policy
Article is made available in accordance with the publisher's policy and may be subject to US copyright law. Please refer to the publisher's site for terms of use.
Terms of use
Metadata
Show full item recordAbstract
Abstract
Weld defect recognition plays an important role in the manufacturing process of large-scale equipment. Traditional methods generally include several serial steps, such as image preprocessing, region segmentation, feature extraction, and type recognition. The results of each step have significant impact on the accuracy of the final defect identification. The convolutional neural network (CNN) has strong pattern recognition ability, which can overcome the above problem. However, there are two problems: one is that the pooling strategy has poor dynamic adaptability and the other is the insufficient feature selection ability. To overcome these problems, we propose a CNN-based weld defect recognition method, which includes an improved pooling strategy and an enhanced feature selection method. According to the characteristics of the weld defect image, an improved pooling strategy that considers the distribution of the pooling region and feature map is introduced. Additionally, in order to enhance the feature selection ability of the CNN, an enhanced feature selection method integrating the ReliefF algorithm with the CNN is proposed. A case study is presented for demonstrating the proposed techniques. The results show that the proposed method has higher accuracy than the traditional CNN method, and establish that the proposed CNN-based method is successfully applied for weld defect recognition.
Date issued
2020-11-16Department
Massachusetts Institute of Technology. Laboratory for Manufacturing and ProductivityPublisher
Springer Berlin Heidelberg