Subspace Clustering with Block Diagonal Sparse Representation
Author(s)
Fang, Xian; Zhang, Ruixun; Li, Zhengxin; Shao, Xiuli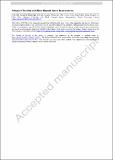
Download11063_2021_10597_ReferencePDF.pdf (1.473Mb)
Publisher Policy
Publisher Policy
Article is made available in accordance with the publisher's policy and may be subject to US copyright law. Please refer to the publisher's site for terms of use.
Terms of use
Metadata
Show full item recordAbstract
Abstract
Structured representation is of remarkable significance in subspace clustering. However, most of the existing subspace clustering algorithms resort to single-structured representation, which may fail to fully capture the essential characteristics of data. To address this issue, a novel multi-structured representation subspace clustering algorithm called block diagonal sparse representation (BDSR) is proposed in this paper. It takes both sparse and block diagonal structured representations into account to obtain the desired affinity matrix. The unified framework is established by integrating the block diagonal prior into the original sparse subspace clustering framework and the resulting optimization problem is iteratively solved by the inexact augmented Lagrange multipliers (IALM). Extensive experiments on both synthetic and real-world datasets well demonstrate the effectiveness and efficiency of the proposed algorithm against the state-of-the-art algorithms.
Date issued
2021-07-23Department
Sloan School of Management. Laboratory for Financial EngineeringPublisher
Springer US
Citation
Fang, Xian, Zhang, Ruixun, Li, Zhengxin and Shao, Xiuli. 2021. "Subspace Clustering with Block Diagonal Sparse Representation."
Version: Author's final manuscript