Teacher improves learning by selecting a training subset
Author(s)
Ma, Y; Nowak, R; Rigollet, P; Zhang, X; Zhu, X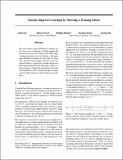
DownloadPublished version (638.8Kb)
Publisher Policy
Publisher Policy
Article is made available in accordance with the publisher's policy and may be subject to US copyright law. Please refer to the publisher's site for terms of use.
Terms of use
Metadata
Show full item recordAbstract
Copyright 2018 by the author(s). We call a learner super-teachable if a teacher can trim down an iid training set while making the learner learn even better. We provide sharp super-teaching guarantees on two learners: the maximum likelihood estimator for the mean of a Gaussian, and the large margin classifier in 1D. For general learners, we provide a mixed-integer nonlinear programming-based algorithm to find a super teaching set. Empirical experiments show that our algorithm is able to find good super-teaching sets for both regression and classification problems.
Date issued
2018Department
Massachusetts Institute of Technology. Department of MathematicsJournal
International Conference on Artificial Intelligence and Statistics, AISTATS 2018
Citation
Ma, Y, Nowak, R, Rigollet, P, Zhang, X and Zhu, X. 2018. "Teacher improves learning by selecting a training subset." International Conference on Artificial Intelligence and Statistics, AISTATS 2018, 84.
Version: Final published version