Optimality of the Plug-in Estimator for Differential Entropy Estimation under Gaussian Convolutions
Author(s)
Goldfeld, Ziv; Greenewald, Kristjan; Weed, Jonathan; Polyanskiy, Yury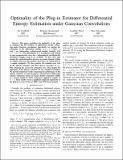
DownloadAccepted version (541.4Kb)
Open Access Policy
Open Access Policy
Creative Commons Attribution-Noncommercial-Share Alike
Terms of use
Metadata
Show full item recordAbstract
© 2019 IEEE. This paper establishes the optimality of the plugin estimator for the problem of differential entropy estimation under Gaussian convolutions. Specifically, we consider the estimation of the differential entropy h(X + Z), where X and Z are independent d-dimensional random variables with Z{\sim}\mathcal{N}( {0,{σ ^2}{{\text{I}}-d}} ). The distribution of X is unknown and belongs to some nonparametric class, but n independently and identically distributed samples from it are available. We first show that despite the regularizing effect of noise, any good estimator (within an additive gap) for this problem must have an exponential in d sample complexity. We then analyze the absolute-error risk of the plug-in estimator and show that it converges as frac{{{c^d}}}{{n }}, thus attaining the parametric estimation rate. This implies the optimality of the plug-in estimator for the considered problem. We provide numerical results comparing the performance of the plug-in estimator to general-purpose (unstructured) differential entropy estimators (based on kernel density estimation (KDE) or k nearest neighbors (kNN) techniques) applied to samples of X + Z. These results reveal a significant empirical superiority of the plug-in to state-of-the-art KDE- and kNN-based methods.
Date issued
2019-09Department
Massachusetts Institute of Technology. Computer Science and Artificial Intelligence Laboratory; Massachusetts Institute of Technology. Department of Electrical Engineering and Computer ScienceJournal
IEEE International Symposium on Information Theory - Proceedings
Publisher
Institute of Electrical and Electronics Engineers (IEEE)
Citation
Goldfeld, Ziv, Greenewald, Kristjan, Weed, Jonathan and Polyanskiy, Yury. 2019. "Optimality of the Plug-in Estimator for Differential Entropy Estimation under Gaussian Convolutions." IEEE International Symposium on Information Theory - Proceedings, 2019-July.
Version: Author's final manuscript