Tensor-Train-based Algorithms for Aggregate State Estimation of Swarms with Interacting Agents
Author(s)
Miculescu, David; Karaman, Sertac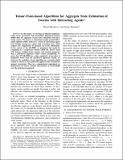
DownloadAccepted version (1.019Mb)
Open Access Policy
Open Access Policy
Creative Commons Attribution-Noncommercial-Share Alike
Terms of use
Metadata
Show full item recordAbstract
© 2020 AACC. In this paper, we develop an efficient implementation of the gas-kinetic (GK) Probability Hypothesis Density (PHD) filter for aggregate swarm state estimation with interacting agents. We borrow a kinetic/mesoscopic partial differential equation (PDE) model of a swarm of interacting agents from biology moving in a plane with a heading state, which requires the computation of integrals up to five dimensions. In the context of the GK-PHD, we propagate this model by computing in a compressed format called the Tensor Train (TT) format, yielding better memory and runtime properties than a grid-based approach. Under certain assumptions, we prove that TT-GK-PHD has a time complexity of an order of magnitude better than the grid-based approach. Finally, we showcase the usefulness of our algorithm on a scenario which cannot be solved via the grid-based approach due to hardware memory constraints. Then in a computational experiment we demonstrate the better runtime and memory of TT-GK-PHD over the grid-based approach.
Date issued
2020-07Department
Massachusetts Institute of Technology. Department of Aeronautics and Astronautics; Massachusetts Institute of Technology. Laboratory for Information and Decision SystemsJournal
Proceedings of the American Control Conference
Publisher
IEEE
Citation
Miculescu, David and Karaman, Sertac. 2020. "Tensor-Train-based Algorithms for Aggregate State Estimation of Swarms with Interacting Agents." Proceedings of the American Control Conference, 2020-July.
Version: Author's final manuscript