A universally optimal multistage accelerated stochastic gradient method
Author(s)
Aybat, NS; Fallah, A; Gürbüzbalaban, M; Ozdaglar, A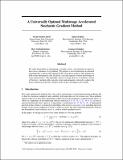
DownloadPublished version (1.419Mb)
Publisher Policy
Publisher Policy
Article is made available in accordance with the publisher's policy and may be subject to US copyright law. Please refer to the publisher's site for terms of use.
Terms of use
Metadata
Show full item recordAbstract
© 2019 Neural information processing systems foundation. All rights reserved. We study the problem of minimizing a strongly convex, smooth function when we have noisy estimates of its gradient. We propose a novel multistage accelerated algorithm that is universally optimal in the sense that it achieves the optimal rate both in the deterministic and stochastic case and operates without knowledge of noise characteristics. The algorithm consists of stages that use a stochastic version of Nesterov's method with a specific restart and parameters selected to achieve the fastest reduction in the bias-variance terms in the convergence rate bounds.
Date issued
2019-12Department
Massachusetts Institute of Technology. Department of Electrical Engineering and Computer ScienceJournal
Advances in Neural Information Processing Systems
Citation
Aybat, NS, Fallah, A, Gürbüzbalaban, M and Ozdaglar, A. 2019. "A universally optimal multistage accelerated stochastic gradient method." Advances in Neural Information Processing Systems, 32.
Version: Final published version