Inductive program synthesis over noisy data
Author(s)
Handa, S; Rinard, MC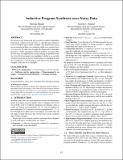
DownloadPublished version (835.3Kb)
Publisher with Creative Commons License
Publisher with Creative Commons License
Creative Commons Attribution
Terms of use
Metadata
Show full item recordAbstract
© 2020 Owner/Author. We present a new framework and associated synthesis algorithms for program synthesis over noisy data, i.e., data that may contain incorrect/corrupted input-output examples. This framework is based on an extension of finite tree automata called state-weighted finite tree automata. We show how to apply this framework to formulate and solve a variety of program synthesis problems over noisy data. Results from our implemented system running on problems from the SyGuS 2018 benchmark suite highlight its ability to successfully synthesize programs in the face of noisy data sets, including the ability to synthesize a correct program even when every input-output example in the data set is corrupted.
Date issued
2020Department
Massachusetts Institute of Technology. Department of Electrical Engineering and Computer ScienceJournal
ESEC/FSE 2020 - Proceedings of the 28th ACM Joint Meeting European Software Engineering Conference and Symposium on the Foundations of Software Engineering
Publisher
ACM
Citation
Handa, S and Rinard, MC. 2020. "Inductive program synthesis over noisy data." ESEC/FSE 2020 - Proceedings of the 28th ACM Joint Meeting European Software Engineering Conference and Symposium on the Foundations of Software Engineering.
Version: Final published version