MemeSequencer: Sparse Matching for Embedding Image Macros
Author(s)
Dubey, Abhimanyu; Moro, Esteban; Cebrian, Manuel; Rahwan, Iyad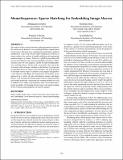
DownloadPublished version (4.399Mb)
Terms of use
Metadata
Show full item recordAbstract
The analysis of the creation, mutation, and propagation of social media content on the Internet is an essential problem in computational social science, affecting areas ranging from marketing to political mobilization. A first step towards understanding the evolution of images online is the analysis of rapidly modifying and propagating memetic imagery or ‘memes’. However, a pitfall in proceeding with such an investigation is the current incapability to produce a robust semantic space for such imagery, capable of understanding differences in Image Macros. In this study, we provide a first step in the systematic study of image evolution on the Internet, by proposing an algorithm based on sparse representations and deep learning to decouple various types of content in such images and produce a rich semantic embedding. We demonstrate the benefits of our approach on a variety of tasks pertaining to memes and Image Macros, such as image clustering, image retrieval, topic prediction and virality prediction, surpassing the existing methods on each.In addition to its utility on quantitative tasks, our method opens up the possibility of obtaining the first large-scale understanding of the evolution and propagation of memetic imagery.
Date issued
2018Department
Massachusetts Institute of Technology. Media LaboratoryPublisher
ACM Press
Citation
Dubey, Abhimanyu, Moro, Esteban, Cebrian, Manuel and Rahwan, Iyad. 2018. "MemeSequencer: Sparse Matching for Embedding Image Macros."
Version: Final published version