Investigating the Effect of the Multiple Comparisons Problem in Visual Analysis
Author(s)
Zgraggen, Emanuel; Zhao, Zheguang; Zeleznik, Robert; Kraska, Tim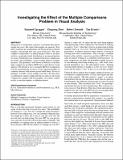
DownloadAccepted version (601.4Kb)
Open Access Policy
Open Access Policy
Creative Commons Attribution-Noncommercial-Share Alike
Terms of use
Metadata
Show full item recordAbstract
© 2018 Association for Computing Machinery. The goal of a visualization system is to facilitate data-driven insight discovery. But what if the insights are spurious? Features or patterns in visualizations can be perceived as relevant insights, even though they may arise from noise. We often compare visualizations to a mental image of what we are interested in: a particular trend, distribution or an unusual pattern. As more visualizations are examined and more comparisons are made, the probability of discovering spurious insights increases. This problem is well-known in Statistics as the multiple comparisons problem (MCP) but overlooked in visual analysis. We present a way to evaluate MCP in visualization tools by measuring the accuracy of user reported insights on synthetic datasets with known ground truth labels. In our experiment, over 60% of user insights were false. We show how a confirmatory analysis approach that accounts for all visual comparisons, insights and non-insights, can achieve similar results as one that requires a validation dataset.
Date issued
2018-04-21Department
Massachusetts Institute of Technology. Department of Electrical Engineering and Computer ScienceJournal
Conference on Human Factors in Computing Systems - Proceedings
Publisher
ACM
Citation
Zgraggen, Emanuel, Zhao, Zheguang, Zeleznik, Robert and Kraska, Tim. 2018. "Investigating the Effect of the Multiple Comparisons Problem in Visual Analysis." Conference on Human Factors in Computing Systems - Proceedings, 2018-April.
Version: Author's final manuscript