Maximum Entropy Fine-Grained Classification
Author(s)
Dubey, Abhimanyu; Gupta, Otkrist; Raskar, Ramesh; Naik, Nikhil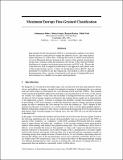
DownloadPublished version (1.243Mb)
Terms of use
Metadata
Show full item recordAbstract
© 2018 Curran Associates Inc..All rights reserved. Fine-Grained Visual Classification (FGVC) is an important computer vision problem that involves small diversity within the different classes, and often requires expert annotators to collect data. Utilizing this notion of small visual diversity, we revisit Maximum-Entropy learning in the context of fine-grained classification, and provide a training routine that maximizes the entropy of the output probability distribution for training convolutional neural networks on FGVC tasks. We provide a theoretical as well as empirical justification of our approach, and achieve state-of-the-art performance across a variety of classification tasks in FGVC, that can potentially be extended to any fine-tuning task. Our method is robust to different hyperparameter values, amount of training data and amount of training label noise and can hence be a valuable tool in many similar problems.
Date issued
2018Department
Massachusetts Institute of Technology. Media LaboratoryCitation
Dubey, Abhimanyu, Gupta, Otkrist, Raskar, Ramesh and Naik, Nikhil. 2018. "Maximum Entropy Fine-Grained Classification."
Version: Final published version