DualSMC: Tunneling Differentiable Filtering and Planning under Continuous POMDPs
Author(s)
Wang, Yunbo; Liu, Bo; Wu, Jiajun; Zhu, Yuke; Du, Simon S; Fei-Fei, Li; Tenenbaum, Joshua B; ... Show more Show less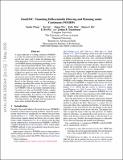
DownloadAccepted version (3.007Mb)
Open Access Policy
Open Access Policy
Creative Commons Attribution-Noncommercial-Share Alike
Terms of use
Metadata
Show full item recordAbstract
© 2020 Inst. Sci. inf., Univ. Defence in Belgrade. All rights reserved. A major difficulty of solving continuous POMDPs is to infer the multi-modal distribution of the unobserved true states and to make the planning algorithm dependent on the perceived uncertainty. We cast POMDP filtering and planning problems as two closely related Sequential Monte Carlo (SMC) processes, one over the real states and the other over the future optimal trajectories, and combine the merits of these two parts in a new model named the DualSMC network. In particular, we first introduce an adversarial particle filter that leverages the adversarial relationship between its internal components. Based on the filtering results, we then propose a planning algorithm that extends the previous SMC planning approach [Piche et al., 2018] to continuous POMDPs with an uncertainty-dependent policy. Crucially, not only can DualSMC handle complex observations such as image input but also it remains highly interpretable. It is shown to be effective in three continuous POMDP domains: the floor positioning domain, the 3D light-dark navigation domain, and a modified Reacher domain.
Date issued
2020Department
Massachusetts Institute of Technology. Department of Brain and Cognitive Sciences; Massachusetts Institute of Technology. Computer Science and Artificial Intelligence LaboratoryJournal
IJCAI International Joint Conference on Artificial Intelligence
Publisher
International Joint Conferences on Artificial Intelligence Organization
Citation
Wang, Yunbo, Liu, Bo, Wu, Jiajun, Zhu, Yuke, Du, Simon S et al. 2020. "DualSMC: Tunneling Differentiable Filtering and Planning under Continuous POMDPs." IJCAI International Joint Conference on Artificial Intelligence, 2021-January.
Version: Author's final manuscript