Deep Learning for Rapid Analysis of Spectroscopic Ellipsometry Data
Author(s)
Li, Yifei; Wu, Yifeng; Yu, Heshan; Takeuchi, Ichiro; Jaramillo, Rafael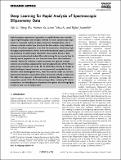
DownloadAdvanced Photonics Research - 2021 - Li - Deep Learning for Rapid Analysis of Spectroscopic Ellipsometry Data.pdf (5.314Mb)
Publisher with Creative Commons License
Publisher with Creative Commons License
Creative Commons Attribution
Additional downloads
Open Access Policy
Open Access Policy
Creative Commons Attribution-Noncommercial-Share Alike
Terms of use
Metadata
Show full item recordAbstract
High-throughput experimental approaches to rapidly develop new materialsrequire high-throughput data analysis methods to match. Spectroscopic ellips-ometry is a powerful method of optical properties characterization, but forunknown materials and/or layer structures the data analysis using traditionalmethods of nonlinear regression is too slow for autonomous, closed-loop, high-throughput experimentation. Herein, three methods (termed spectral, piecewise,and pointwise) of spectroscopic ellipsometry data analysis based on deeplearning are introduced and studied. After initial training, the incremental time forinferring optical properties can be a thousand times faster than traditionalmethods. Results for multilayer sample structures with optically isotropicmaterials are presented, appropriate for high-throughput studies of thinfilms ofphase-change materials such as Ge─Sb─Te (GST) alloys. Results for studies onhighly birefringent layered materials are also presented, exemplified by thetransition metal dichalcogenide MoS2. How the materials under test and theexperimental objectives may guide the choice of analysis methods are discussed.The utility of our approach is demonstrated by analyzing data measured on acomposition spread of Ge─Sb─Te phase-change alloys containing 177 distinctcompositions, and identifying the composition with optimal phase-changefigureof merit in only 1.4 s of analysis time.
Date issued
2021-09-23Department
Massachusetts Institute of Technology. Department of Materials Science and EngineeringJournal
Advanced Photonics Research
Publisher
Wiley
Citation
Li, Yifei, Wu, Yifeng, Yu, Heshan, Takeuchi, Ichiro and Jaramillo, Rafael. 2021. "Deep Learning for Rapid Analysis of Spectroscopic Ellipsometry Data." Advanced Photonics Research, 2 (12).
Version: Final published version
ISSN
2699-9293
2699-9293