Flow Control in Wings and Discovery of Novel Approaches via Deep Reinforcement Learning
Author(s)
Vinuesa, Ricardo; Lehmkuhl, Oriol; Lozano-Durán, Adrian; Rabault, Jean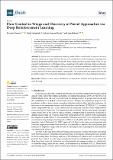
Downloadfluids-07-00062.pdf (5.982Mb)
Publisher with Creative Commons License
Publisher with Creative Commons License
Creative Commons Attribution
Terms of use
Metadata
Show full item recordAbstract
In this review, we summarize existing trends of flow control used to improve the aerodynamic efficiency of wings. We first discuss active methods to control turbulence, starting with flat-plate geometries and building towards the more complicated flow around wings. Then, we discuss active approaches to control separation, a crucial aspect towards achieving a high aerodynamic efficiency. Furthermore, we highlight methods relying on turbulence simulation, and discuss various levels of modeling. Finally, we thoroughly revise data-driven methods and their application to flow control, and focus on deep reinforcement learning (DRL). We conclude that this methodology has the potential to discover novel control strategies in complex turbulent flows of aerodynamic relevance.
Date issued
2022-02-01Department
Massachusetts Institute of Technology. Department of Aeronautics and AstronauticsJournal
Fluids
Publisher
Multidisciplinary Digital Publishing Institute
Citation
Vinuesa, R.; Lehmkuhl, O.; Lozano-Durán, A.; Rabault, J. Flow Control in Wings and Discovery of Novel Approaches via Deep Reinforcement Learning. Fluids 7 (2): 62 (2022)
Version: Final published version
ISSN
2311-5521