Data-driven materials research enabled by natural language processing and information extraction
Author(s)
Olivetti, Elsa A; Cole, Jacqueline M; Kim, Edward; Kononova, Olga; Ceder, Gerbrand; Han, Thomas Yong-Jin; Hiszpanski, Anna M; ... Show more Show less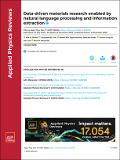
DownloadPublished version (3.244Mb)
Publisher Policy
Publisher Policy
Article is made available in accordance with the publisher's policy and may be subject to US copyright law. Please refer to the publisher's site for terms of use.
Terms of use
Metadata
Show full item recordAbstract
© 2020 Author(s). Given the emergence of data science and machine learning throughout all aspects of society, but particularly in the scientific domain, there is increased importance placed on obtaining data. Data in materials science are particularly heterogeneous, based on the significant range in materials classes that are explored and the variety of materials properties that are of interest. This leads to data that range many orders of magnitude, and these data may manifest as numerical text or image-based information, which requires quantitative interpretation. The ability to automatically consume and codify the scientific literature across domains - enabled by techniques adapted from the field of natural language processing - therefore has immense potential to unlock and generate the rich datasets necessary for data science and machine learning. This review focuses on the progress and practices of natural language processing and text mining of materials science literature and highlights opportunities for extracting additional information beyond text contained in figures and tables in articles. We discuss and provide examples for several reasons for the pursuit of natural language processing for materials, including data compilation, hypothesis development, and understanding the trends within and across fields. Current and emerging natural language processing methods along with their applications to materials science are detailed. We, then, discuss natural language processing and data challenges within the materials science domain where future directions may prove valuable.
Date issued
2020Department
Massachusetts Institute of Technology. Department of Materials Science and EngineeringJournal
Applied Physics Reviews
Publisher
AIP Publishing
Citation
Olivetti, Elsa A, Cole, Jacqueline M, Kim, Edward, Kononova, Olga, Ceder, Gerbrand et al. 2020. "Data-driven materials research enabled by natural language processing and information extraction." Applied Physics Reviews, 7 (4).
Version: Final published version