Confident Learning: Estimating Uncertainty in Dataset Labels
Author(s)
Northcutt, Curtis; Jiang, Lu; Chuang, Isaac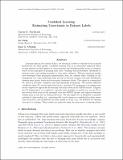
Download12125-Article (PDF)-26691-1-10-20210414.pdf (6.934Mb)
Publisher Policy
Publisher Policy
Article is made available in accordance with the publisher's policy and may be subject to US copyright law. Please refer to the publisher's site for terms of use.
Terms of use
Metadata
Show full item recordAbstract
<jats:p>Learning exists in the context of data, yet notions of confidence typically focus on model predictions, not label quality. Confident learning (CL) is an alternative approach which focuses instead on label quality by characterizing and identifying label errors in datasets, based on the principles of pruning noisy data, counting with probabilistic thresholds to estimate noise, and ranking examples to train with confidence. Whereas numerous studies have developed these principles independently, here, we combine them, building on the assumption of a class-conditional noise process to directly estimate the joint distribution between noisy (given) labels and uncorrupted (unknown) labels. This results in a generalized CL which is provably consistent and experimentally performant. We present sufficient conditions where CL exactly finds label errors, and show CL performance exceeding seven recent competitive approaches for learning with noisy labels on the CIFAR dataset. Uniquely, the CL framework is not coupled to a specific data modality or model (e.g., we use CL to find several label errors in the presumed error-free MNIST dataset and improve sentiment classification on text data in Amazon Reviews). We also employ CL on ImageNet to quantify ontological class overlap (e.g., estimating 645 missile images are mislabeled as their parent class projectile), and moderately increase model accuracy (e.g., for ResNet) by cleaning data prior to training. These results are replicable using the open-source cleanlab release.</jats:p>
Date issued
2021Department
Massachusetts Institute of Technology. Department of Electrical Engineering and Computer ScienceJournal
Journal of Artificial Intelligence Research
Publisher
AI Access Foundation
Citation
Northcutt, Curtis, Jiang, Lu and Chuang, Isaac. 2021. "Confident Learning: Estimating Uncertainty in Dataset Labels." Journal of Artificial Intelligence Research, 70.
Version: Final published version