Hogwild!-Gibbs can be panaccurate
Author(s)
Daskalakis, C; Dikkala, N; Jayanti, S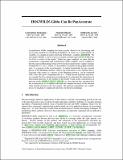
DownloadPublished version (374.6Kb)
Publisher Policy
Publisher Policy
Article is made available in accordance with the publisher's policy and may be subject to US copyright law. Please refer to the publisher's site for terms of use.
Terms of use
Metadata
Show full item recordAbstract
© 2018 Curran Associates Inc..All rights reserved. Asynchronous Gibbs sampling has been recently shown to be fast-mixing and an accurate method for estimating probabilities of events on a small number of variables of a graphical model satisfying Dobrushin's condition [DSOR16]. We investigate whether it can be used to accurately estimate expectations of functions of all the variables of the model. Under the same condition, we show that the synchronous (sequential) and asynchronous Gibbs samplers can be coupled so that the expected Hamming distance between their (multivariate) samples remains bounded by O(τ log n), where n is the number of variables in the graphical model, and τ is a measure of the asynchronicity. A similar bound holds for any constant power of the Hamming distance. Hence, the expectation of any function that is Lipschitz with respect to a power of the Hamming distance, can be estimated with a bias that grows logarithmically in n. Going beyond Lipschitz functions, we consider the bias arising from asynchronicity in estimating the expectation of polynomial functions of all variables in the model. Using recent concentration of measure results [DDK17, GLP17, GSS18], we show that the bias introduced by the asynchronicity is of smaller order than the standard deviation of the function value already present in the true model. We perform experiments on a multiprocessor machine to empirically illustrate our theoretical findings.
Date issued
2018-01-01Department
Massachusetts Institute of Technology. Department of Electrical Engineering and Computer Science; Massachusetts Institute of Technology. Computer Science and Artificial Intelligence LaboratoryJournal
Advances in Neural Information Processing Systems
Citation
Daskalakis, C, Dikkala, N and Jayanti, S. 2018. "Hogwild!-Gibbs can be panaccurate." Advances in Neural Information Processing Systems, 2018-December.
Version: Final published version