Generative modeling of single-cell time series with PRESCIENT enables prediction of cell trajectories with interventions
Author(s)
Yeo, Grace Hui Ting; Saksena, Sachit D; Gifford, David K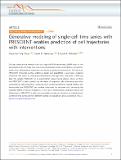
DownloadPublished version (3.386Mb)
Publisher with Creative Commons License
Publisher with Creative Commons License
Creative Commons Attribution
Terms of use
Metadata
Show full item recordAbstract
Abstract
Existing computational methods that use single-cell RNA-sequencing (scRNA-seq) for cell fate prediction do not model how cells evolve stochastically and in physical time, nor can they predict how differentiation trajectories are altered by proposed interventions. We introduce PRESCIENT (Potential eneRgy undErlying Single Cell gradIENTs), a generative modeling framework that learns an underlying differentiation landscape from time-series scRNA-seq data. We validate PRESCIENT on an experimental lineage tracing dataset, where we show that PRESCIENT is able to predict the fate biases of progenitor cells in hematopoiesis when accounting for cell proliferation, improving upon the best-performing existing method. We demonstrate how PRESCIENT can simulate trajectories for perturbed cells, recovering the expected effects of known modulators of cell fate in hematopoiesis and pancreatic β cell differentiation. PRESCIENT is able to accommodate complex perturbations of multiple genes, at different time points and from different starting cell populations, and is available at t https://
github.com/gifford-lab/prescient.
Date issued
2021Department
Massachusetts Institute of Technology. Computer Science and Artificial Intelligence Laboratory; Massachusetts Institute of Technology. Computational and Systems Biology Program; Massachusetts Institute of Technology. Department of Electrical Engineering and Computer Science; Massachusetts Institute of Technology. Department of Biological EngineeringJournal
Nature Communications
Publisher
Springer Science and Business Media LLC
Citation
Yeo, Grace Hui Ting, Saksena, Sachit D and Gifford, David K. 2021. "Generative modeling of single-cell time series with PRESCIENT enables prediction of cell trajectories with interventions." Nature Communications, 12 (1).
Version: Final published version