Machine learning based CRISPR gRNA design for therapeutic exon skipping
Author(s)
Louie, Wilson; Shen, Max W; Tahiry, Zakir; Zhang, Sophia; Worstell, Daniel; Cassa, Christopher A; Sherwood, Richard I; Gifford, David K; ... Show more Show less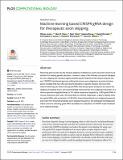
DownloadPublished version (2.526Mb)
Publisher with Creative Commons License
Publisher with Creative Commons License
Creative Commons Attribution
Terms of use
Metadata
Show full item recordAbstract
© 2021 Louie et al. This is an open access article distributed under the terms of the Creative Commons Attribution License, which permits unrestricted use, distribution, and reproduction in any medium, provided the original author and source are credited. Restoring gene function by the induced skipping of deleterious exons has been shown to be effective for treating genetic disorders. However, many of the clinically successful therapies for exon skipping are transient oligonucleotide-based treatments that require frequent dosing. CRISPR-Cas9 based genome editing that causes exon skipping is a promising therapeutic modality that may offer permanent alleviation of genetic disease. We show that machine learning can select Cas9 guide RNAs that disrupt splice acceptors and cause the skipping of targeted exons. We experimentally measured the exon skipping frequencies of a diverse genome-integrated library of 791 splice sequences targeted by 1,063 guide RNAs in mouse embryonic stem cells. We found that our method, SkipGuide, is able to identify effective guide RNAs with a precision of 0.68 (50% threshold predicted exon skipping frequency) and 0.93 (70% threshold predicted exon skipping frequency). We anticipate that SkipGuide will be useful for selecting guide RNA candidates for evaluation of CRISPR-Cas9-mediated exon skipping therapy.
Date issued
2021Department
Massachusetts Institute of Technology. Computer Science and Artificial Intelligence Laboratory; Massachusetts Institute of Technology. Department of Electrical Engineering and Computer Science; Massachusetts Institute of Technology. Computational and Systems Biology Program; Massachusetts Institute of Technology. Department of Biological EngineeringJournal
PLoS Computational Biology
Publisher
Public Library of Science (PLoS)
Citation
Louie, Wilson, Shen, Max W, Tahiry, Zakir, Zhang, Sophia, Worstell, Daniel et al. 2021. "Machine learning based CRISPR gRNA design for therapeutic exon skipping." PLoS Computational Biology, 17 (1).
Version: Final published version