Technical Note—Two-Stage Sample Robust Optimization
Author(s)
Bertsimas, Dimitris; Shtern, Shimrit; Sturt, Bradley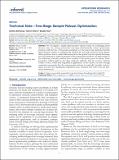
DownloadAccepted version (1.368Mb)
Open Access Policy
Open Access Policy
Creative Commons Attribution-Noncommercial-Share Alike
Terms of use
Metadata
Show full item recordAbstract
<jats:p> In “Two-Stage Sample Robust Optimization,” Bertsimas, Shtern, and Sturt investigate a simple approximation scheme, based on overlapping linear decision rules, for solving data-driven two-stage distributionally robust optimization problems with the type-infinity Wasserstein ambiguity set. Their main result establishes that this approximation scheme is asymptotically optimal for two-stage stochastic linear optimization problems; that is, under mild assumptions, the optimal cost and optimal first-stage decisions obtained by approximating the robust optimization problem converge to those of the underlying stochastic problem as the number of data points grows to infinity. These guarantees notably apply to two-stage stochastic problems that do not have relatively complete recourse, which arise frequently in applications. In this context, the authors show through numerical experiments that the approximation scheme is practically tractable and produces decisions that significantly outperform those obtained from state-of-the-art data-driven alternatives. </jats:p>
Date issued
2022Department
Massachusetts Institute of Technology. Operations Research CenterJournal
Operations Research
Publisher
Institute for Operations Research and the Management Sciences (INFORMS)
Citation
Bertsimas, Dimitris, Shtern, Shimrit and Sturt, Bradley. 2022. "Technical Note—Two-Stage Sample Robust Optimization." Operations Research, 70 (1).
Version: Author's final manuscript