Linear Program-Based Approximation for Personalized Reserve Prices
Author(s)
Derakhshan, Mahsa; Golrezaei, Negin; Paes Leme, Renato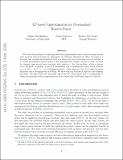
DownloadAccepted version (573.8Kb)
Open Access Policy
Open Access Policy
Creative Commons Attribution-Noncommercial-Share Alike
Terms of use
Metadata
Show full item recordAbstract
<jats:p> We study the problem of computing data-driven personalized reserve prices in eager second price auctions without having any assumption on valuation distributions. Here, the input is a data set that contains the submitted bids of n buyers in a set of auctions, and the problem is to return personalized reserve prices r that maximize the revenue earned on these auctions by running eager second price auctions with reserve r. For this problem, which is known to be NP complete, we present a novel linear program (LP) formulation and a rounding procedure, which achieves a 0.684 approximation. This improves over the [Formula: see text]-approximation algorithm from Roughgarden and Wang. We show that our analysis is tight for this rounding procedure. We also bound the integrality gap of the LP, which shows that it is impossible to design an algorithm that yields an approximation factor larger than 0.828 with respect to this LP. </jats:p><jats:p> This paper was accepted by Chung Piaw Teo, Management Science Special Section on Data-Driven Prescriptive Analytics. </jats:p>
Date issued
2022Department
Sloan School of ManagementJournal
Management Science
Publisher
Institute for Operations Research and the Management Sciences (INFORMS)
Citation
Derakhshan, Mahsa, Golrezaei, Negin and Paes Leme, Renato. 2022. "Linear Program-Based Approximation for Personalized Reserve Prices." Management Science, 68 (3).
Version: Author's final manuscript