Causal Structure Learning: A Combinatorial Perspective
Author(s)
Squires, Chandler; Uhler, Caroline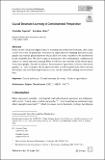
Download10208_2022_Article_9581.pdf (829.3Kb)
Publisher with Creative Commons License
Publisher with Creative Commons License
Creative Commons Attribution
Terms of use
Metadata
Show full item recordAbstract
Abstract
In this review, we discuss approaches for learning causal structure from data, also called causal discovery. In particular, we focus on approaches for learning directed acyclic graphs and various generalizations which allow for some variables to be unobserved in the available data. We devote special attention to two fundamental combinatorial aspects of causal structure learning. First, we discuss the structure of the search space over causal graphs. Second, we discuss the structure of equivalence classes over causal graphs, i.e., sets of graphs which represent what can be learned from observational data alone, and how these equivalence classes can be refined by adding interventional data.
Date issued
2022-08-01Department
Massachusetts Institute of Technology. Department of Electrical Engineering and Computer Science; Massachusetts Institute of Technology. Institute for Data, Systems, and SocietyPublisher
Springer US
Citation
Squires, Chandler and Uhler, Caroline. 2022. "Causal Structure Learning: A Combinatorial Perspective."
Version: Final published version