Machine-learning potentials for crystal defects
Author(s)
Freitas, Rodrigo; Cao, Yifan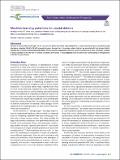
Download43579_2022_Article_221.pdf (3.476Mb)
Publisher with Creative Commons License
Publisher with Creative Commons License
Creative Commons Attribution
Terms of use
Metadata
Show full item recordAbstract
Abstract
Decades of advancements in strategies for the calculation of atomic interactions have culminated in a class of methods known as machine-learning interatomic potentials (MLIAPs). MLIAPs dramatically widen the spectrum of materials systems that can be simulated with high physical fidelity, including their microstructural evolution and kinetics. This framework, in conjunction with cross-scale simulations and in silico microscopy, is poised to bring a paradigm shift to the field of atomistic simulations of materials. In this prospective article we summarize recent progress in the application of MLIAPs to crystal defects.
Graphical abstract
Date issued
2022-08-12Department
Massachusetts Institute of Technology. Department of Materials Science and EngineeringPublisher
Springer International Publishing
Citation
Freitas, Rodrigo and Cao, Yifan. 2022. "Machine-learning potentials for crystal defects."
Version: Final published version