Polynomial-time algorithms for multimarginal optimal transport problems with structure
Author(s)
Altschuler, Jason M.; Boix-Adserà, Enric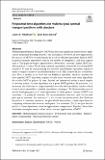
Download10107_2022_Article_1868.pdf (1.885Mb)
Publisher with Creative Commons License
Publisher with Creative Commons License
Creative Commons Attribution
Terms of use
Metadata
Show full item recordAbstract
Abstract
Multimarginal Optimal Transport (MOT) has attracted significant interest due to applications in machine learning, statistics, and the sciences. However, in most applications, the success of MOT is severely limited by a lack of efficient algorithms. Indeed, MOT in general requires exponential time in the number of marginals k and their support sizes n. This paper develops a general theory about what “structure” makes MOT solvable in
$$\mathrm {poly}(n,k)$$
poly
(
n
,
k
)
time. We develop a unified algorithmic framework for solving MOT in
$$\mathrm {poly}(n,k)$$
poly
(
n
,
k
)
time by characterizing the structure that different algorithms require in terms of simple variants of the dual feasibility oracle. This framework has several benefits. First, it enables us to show that the Sinkhorn algorithm, which is currently the most popular MOT algorithm, requires strictly more structure than other algorithms do to solve MOT in
$$\mathrm {poly}(n,k)$$
poly
(
n
,
k
)
time. Second, our framework makes it much simpler to develop
$$\mathrm {poly}(n,k)$$
poly
(
n
,
k
)
time algorithms for a given MOT problem. In particular, it is necessary and sufficient to (approximately) solve the dual feasibility oracle—which is much more amenable to standard algorithmic techniques. We illustrate this ease-of-use by developing
$$\mathrm {poly}(n,k)$$
poly
(
n
,
k
)
-time algorithms for three general classes of MOT cost structures: (1) graphical structure; (2) set-optimization structure; and (3) low-rank plus sparse structure. For structure (1), we recover the known result that Sinkhorn has
$$\mathrm {poly}(n,k)$$
poly
(
n
,
k
)
runtime; moreover, we provide the first
$$\mathrm {poly}(n,k)$$
poly
(
n
,
k
)
time algorithms for computing solutions that are exact and sparse. For structures (2)-(3), we give the first
$$\mathrm {poly}(n,k)$$
poly
(
n
,
k
)
time algorithms, even for approximate computation. Together, these three structures encompass many—if not most—current applications of MOT.
Date issued
2022-08-16Department
Massachusetts Institute of Technology. Laboratory for Information and Decision SystemsPublisher
Springer Berlin Heidelberg
Citation
Altschuler, Jason M. and Boix-Adserà, Enric. 2022. "Polynomial-time algorithms for multimarginal optimal transport problems with structure."
Version: Final published version