Distributional conformal prediction
Author(s)
Chernozhukov, Victor; Wüthrich, Kaspar; Zhu, Yinchu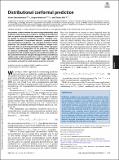
DownloadPublished version (871.9Kb)
Publisher Policy
Publisher Policy
Article is made available in accordance with the publisher's policy and may be subject to US copyright law. Please refer to the publisher's site for terms of use.
Terms of use
Metadata
Show full item recordAbstract
<jats:title>Significance</jats:title>
<jats:p>Prediction problems are important in many contexts. Examples include cross-sectional prediction, time series forecasting, counterfactual prediction and synthetic controls, and individual treatment effect prediction. We develop a prediction method that works in conjunction with many powerful classical methods (e.g., conventional quantile regression) as well as modern high-dimensional methods for estimating conditional distributions (e.g., quantile neural networks). Unlike many existing prediction approaches, our method is valid conditional on the observed predictors and efficient under some conditions. Importantly, our method is also robust; it exhibits unconditional coverage guarantees under model misspecification, under overfitting, and with time series data.</jats:p>
Date issued
2021Department
Massachusetts Institute of Technology. Department of Economics; Statistics and Data Science Center (Massachusetts Institute of Technology)Journal
Proceedings of the National Academy of Sciences of the United States of America
Publisher
Proceedings of the National Academy of Sciences
Citation
Chernozhukov, Victor, Wüthrich, Kaspar and Zhu, Yinchu. 2021. "Distributional conformal prediction." Proceedings of the National Academy of Sciences of the United States of America, 118 (48).
Version: Final published version