Rapid mechanical property prediction and <i>de novo</i> design of three-dimensional spider webs through graph and GraphPerceiver neural networks
Author(s)
Lu, Wei; Yang, Zhenze; Buehler, Markus J.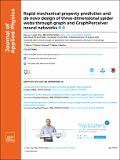
Download5.0097589.pdf (4.053Mb)
Publisher with Creative Commons License
Publisher with Creative Commons License
Creative Commons Attribution
Terms of use
Metadata
Show full item recordAbstract
<jats:p> Spider webs feature advanced structural performance due to the evolutionary success of over more than 3 × 10<jats:sup>9</jats:sup> years, including lightweight design and exceptional mechanical properties. Spider webs are appealing for bio-inspired design since web designs serve multiple functions including mechanical protection and prey catching. However, high computational cost and limited quantified web properties render extensive spider web studies challenging in part due to the high structural complexity and randomness of fiber arrangements in 3D webs. Here, we report a computational method to relate spider web graph microstructures to effective mechanical properties, focusing on strength and toughness, and upscaling from the microscopic to the mesoscale level. The new computational framework uses deep neural networks, trained on graph-structured Cyrtophora citricola spider web mechanical data, in order to capture complex cross-scale structural relationships. Three different models are developed and compared. First, two Graph Neural Network (GNN) models, a Graph Convolutional Network, and a Principal Neighborhood Aggregation method. Second, a GraphPerceiver transformer model that is fed similar input data as provided to the GNN approach but within a natural language modeling context using self-attention mechanisms. The GraphPerceiver model can achieve similar performance as the GNN model, offering added flexibility for building deep learning models of diverse hierarchical biological materials. As an application of the model, we propose a computational optimization tool for synthetic web design that is used to generate synthetic, de novo spider web architectures. Finally, multi-objective optimization enables us to discover web structures that meet specific mechanical properties as design objectives. </jats:p>
Date issued
2022-08-21Department
Massachusetts Institute of Technology. Department of Civil and Environmental Engineering; Massachusetts Institute of Technology. Department of Materials Science and Engineering; Massachusetts Institute of Technology. Department of Mechanical Engineering; Massachusetts Institute of Technology. Laboratory for Atomistic and Molecular MechanicsPublisher
AIP Publishing
Citation
Lu, Wei, Yang, Zhenze and Buehler, Markus J. 2022. "Rapid mechanical property prediction and <i>de novo</i> design of three-dimensional spider webs through graph and GraphPerceiver neural networks." 132 (7).
Version: Final published version
ISSN
0021-8979
1089-7550