Hierarchical Reinforcement Learning for Open-Domain Dialog
Author(s)
Saleh, Abdelrhman; Jaques, Natasha; Ghandeharioun, Asma; Shen, Judy; Picard, Rosalind W.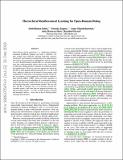
DownloadAccepted version (1.517Mb)
Open Access Policy
Open Access Policy
Creative Commons Attribution-Noncommercial-Share Alike
Terms of use
Metadata
Show full item recordAbstract
<jats:p>Open-domain dialog generation is a challenging problem; maximum likelihood training can lead to repetitive outputs, models have difficulty tracking long-term conversational goals, and training on standard movie or online datasets may lead to the generation of inappropriate, biased, or offensive text. Reinforcement Learning (RL) is a powerful framework that could potentially address these issues, for example by allowing a dialog model to optimize for reducing toxicity and repetitiveness. However, previous approaches which apply RL to open-domain dialog generation do so at the word level, making it difficult for the model to learn proper credit assignment for long-term conversational rewards. In this paper, we propose a novel approach to hierarchical reinforcement learning (HRL), VHRL, which uses policy gradients to tune the utterance-level embedding of a variational sequence model. This hierarchical approach provides greater flexibility for learning long-term, conversational rewards. We use self-play and RL to optimize for a set of human-centered conversation metrics, and show that our approach provides significant improvements – in terms of both human evaluation and automatic metrics – over state-of-the-art dialog models, including Transformers.</jats:p>
Date issued
2020Department
Program in Media Arts and Sciences (Massachusetts Institute of Technology)Journal
Proceedings of the AAAI Conference on Artificial Intelligence
Publisher
Association for the Advancement of Artificial Intelligence (AAAI)
Citation
Saleh, Abdelrhman, Jaques, Natasha, Ghandeharioun, Asma, Shen, Judy and Picard, Rosalind. 2020. "Hierarchical Reinforcement Learning for Open-Domain Dialog." Proceedings of the AAAI Conference on Artificial Intelligence, 34 (05).
Version: Author's final manuscript