Modeling Atomistic Dynamic Fracture Mechanisms Using a Progressive Transformer Diffusion Model
Author(s)
Buehler, Markus J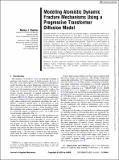
DownloadPublished version (1.595Mb)
Publisher Policy
Publisher Policy
Article is made available in accordance with the publisher's policy and may be subject to US copyright law. Please refer to the publisher's site for terms of use.
Terms of use
Metadata
Show full item recordAbstract
<jats:title>Abstract</jats:title><jats:p>Dynamic fracture is an important area of materials analysis, assessing the atomic-level mechanisms by which materials fail over time. Here, we focus on brittle materials failure and show that an atomistically derived progressive transformer diffusion machine learning model can effectively describe the dynamics of fracture, capturing important aspects such as crack dynamics, instabilities, and initiation mechanisms. Trained on a small dataset of atomistic simulations, the model generalizes well and offers a rapid assessment of dynamic fracture mechanisms for complex geometries, expanding well beyond the original set of atomistic simulation results. Various validation cases, progressively more distinct from the data used for training, are presented and analyzed. The validation cases feature distinct geometric details, including microstructures generated by a generative neural network used here to identify novel bio-inspired material designs for mechanical performance. For all cases, the model performs well and captures key aspects of material failure.</jats:p>
Date issued
2022Department
Massachusetts Institute of Technology. Department of Civil and Environmental EngineeringJournal
Journal of Applied Mechanics
Publisher
ASME International
Citation
Buehler, Markus J. 2022. "Modeling Atomistic Dynamic Fracture Mechanisms Using a Progressive Transformer Diffusion Model." Journal of Applied Mechanics, 89 (12).
Version: Final published version