Distributionally Robust Linear and Discrete Optimization with Marginals
Author(s)
Chen, Louis; Ma, Will; Natarajan, Karthik; Simchi-Levi, David; Yan, Zhenzhen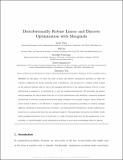
DownloadSubmitted version (727.2Kb)
Open Access Policy
Open Access Policy
Creative Commons Attribution-Noncommercial-Share Alike
Terms of use
Metadata
Show full item recordAbstract
<jats:p> In optimization problems, decisions are often made in the face of uncertainty that might arise in the form of random costs or benefits. In “Distributionally Robust Linear and Discrete Optimization with Marginals,” Louis Chen, Will Ma, Karthik Natarajan, David Simchi-Levi, and Zhenzhen Yan study a robust bound of linear and discrete optimization problems in which the objective coefficients are random and the set of admissible joint distributions is assumed to be specified only up to the marginals. They provide a primal-dual formulation for this problem, and in the process, unify existing results with new results. They establish NP-hardness of computing the bound for general polytopes and identify two sufficient conditions—one based on a dual formulation and one based on sublattices that provide a class of polytopes where the robust bounds are efficiently computable. </jats:p>
Date issued
2022Department
Massachusetts Institute of Technology. Department of Civil and Environmental EngineeringJournal
Operations Research
Publisher
Institute for Operations Research and the Management Sciences (INFORMS)
Citation
Chen, Louis, Ma, Will, Natarajan, Karthik, Simchi-Levi, David and Yan, Zhenzhen. 2022. "Distributionally Robust Linear and Discrete Optimization with Marginals." Operations Research, 70 (3).
Version: Author's final manuscript