Reinforcement Learning for Mixed Autonomy Intersections
Author(s)
Yan, Zhongxia; Wu, Cathy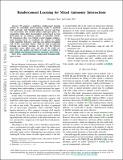
DownloadAccepted version (2.084Mb)
Open Access Policy
Open Access Policy
Creative Commons Attribution-Noncommercial-Share Alike
Terms of use
Metadata
Show full item recordAbstract
We propose a model-free reinforcement learning method for controlling mixed
autonomy traffic in simulated traffic networks with through-traffic-only
two-way and four-way intersections. Our method utilizes multi-agent policy
decomposition which allows decentralized control based on local observations
for an arbitrary number of controlled vehicles. We demonstrate that, even
without reward shaping, reinforcement learning learns to coordinate the
vehicles to exhibit traffic signal-like behaviors, achieving near-optimal
throughput with 33-50% controlled vehicles. With the help of multi-task
learning and transfer learning, we show that this behavior generalizes across
inflow rates and size of the traffic network. Our code, models, and videos of
results are available at
https://github.com/ZhongxiaYan/mixed_autonomy_intersections.
Date issued
2021Department
Massachusetts Institute of Technology. Department of Civil and Environmental EngineeringJournal
2021 IEEE International Intelligent Transportation Systems Conference (ITSC)
Publisher
IEEE
Citation
Yan, Zhongxia and Wu, Cathy. 2021. "Reinforcement Learning for Mixed Autonomy Intersections." 2021 IEEE International Intelligent Transportation Systems Conference (ITSC).
Version: Author's final manuscript