Semantic projection recovers rich human knowledge of multiple object features from word embeddings
Author(s)
Grand, Gabriel; Blank, Idan Asher; Pereira, Francisco; Fedorenko, Evelina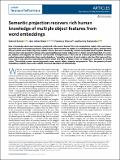
DownloadAccepted version (2.561Mb)
Open Access Policy
Open Access Policy
Creative Commons Attribution-Noncommercial-Share Alike
Terms of use
Metadata
Show full item recordAbstract
How is knowledge about word meaning represented in the mental lexicon? Current computational models infer word meanings from lexical co-occurrence patterns. They learn to represent words as vectors in a multidimensional space, wherein words that are used in more similar linguistic contexts-that is, are more semantically related-are located closer together. However, whereas inter-word proximity captures only overall relatedness, human judgements are highly context dependent. For example, dolphins and alligators are similar in size but differ in dangerousness. Here, we use a domain-general method to extract context-dependent relationships from word embeddings: 'semantic projection' of word-vectors onto lines that represent features such as size (the line connecting the words 'small' and 'big') or danger ('safe' to 'dangerous'), analogous to 'mental scales'. This method recovers human judgements across various object categories and properties. Thus, the geometry of word embeddings explicitly represents a wealth of context-dependent world knowledge.
Date issued
2022Department
Massachusetts Institute of Technology. Department of Brain and Cognitive SciencesJournal
Nature Human Behaviour
Publisher
Springer Science and Business Media LLC
Citation
Grand, Gabriel, Blank, Idan Asher, Pereira, Francisco and Fedorenko, Evelina. 2022. "Semantic projection recovers rich human knowledge of multiple object features from word embeddings." Nature Human Behaviour, 6 (7).
Version: Author's final manuscript