Can machine learning identify childhood characteristics that predict future development of bipolar disorder a decade later?
Author(s)
Uchida, Mai; Bukhari, Qasim; DiSalvo, Maura; Green, Allison; Serra, Giulia; Hutt Vater, Chloe; Ghosh, Satrajit S; Faraone, Stephen V; Gabrieli, John DE; Biederman, Joseph; ... Show more Show less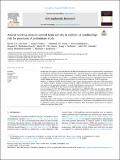
DownloadAccepted version (766.3Kb)
Publisher with Creative Commons License
Publisher with Creative Commons License
Creative Commons Attribution
Terms of use
Metadata
Show full item recordAbstract
Early identification of bipolar disorder may provide appropriate support and treatment, however there is no current evidence for statistically predicting whether a child will develop bipolar disorder. Machine learning methods offer an opportunity for developing empirically-based predictors of bipolar disorder. This study examined whether bipolar disorder can be predicted using clinical data and machine learning algorithms. 492 children, ages 6-18 at baseline, were recruited from longitudinal case-control family studies. Participants were assessed at baseline, then followed-up after 10 years. In addition to sociodemographic data, children were assessed with psychometric scales, structured diagnostic interviews, and cognitive and social functioning assessments. Using the Balanced Random Forest algorithm, we examined whether the diagnostic outcome of full or subsyndromal bipolar disorder could be predicted from baseline data. 45 children (10%) developed bipolar disorder at follow-up. The model predicted subsequent bipolar disorder with 75% sensitivity, 76% specificity, and an Area Under the Receiver Operating Characteristics of 75%. Predictors best differentiating between children who did or did not develop bipolar disorder were the Child Behavioral Checklist Externalizing and Internalizing behaviors, the Child Behavioral Checklist Total t-score, problematic school functions indexed through the Child Behavioral Checklist School Competence scale, and the Child Behavioral Checklist Anxiety/Depression and Aggression scales. Our study provides the first quantitative model to predict bipolar disorder. Longitudinal prediction may help clinicians assess children with emergent psychopathology for future risk of bipolar disorder, an area of clinical and scientific importance. Machine learning algorithms could be implemented to alert clinicians to risk for bipolar disorder.
Date issued
2022Department
Massachusetts Institute of Technology. Department of Brain and Cognitive SciencesJournal
Journal of Psychiatric Research
Publisher
Elsevier BV
Citation
Uchida, Mai, Bukhari, Qasim, DiSalvo, Maura, Green, Allison, Serra, Giulia et al. 2022. "Can machine learning identify childhood characteristics that predict future development of bipolar disorder a decade later?." Journal of Psychiatric Research, 156.
Version: Author's final manuscript