Three-dimensional nanoscale reduced-angle ptycho-tomographic imaging with deep learning (RAPID)
Author(s)
Wu, Ziling; Kang, Iksung; Yao, Yudong; Jiang, Yi; Deng, Junjing; Klug, Jeffrey; Vogt, Stefan; Barbastathis, George; ... Show more Show less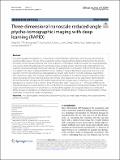
Download43593_2022_Article_37.pdf (2.816Mb)
Publisher with Creative Commons License
Publisher with Creative Commons License
Creative Commons Attribution
Terms of use
Metadata
Show full item recordAbstract
Abstract
X-ray ptychographic tomography is a nondestructive method for three dimensional (3D) imaging with nanometer-sized resolvable features. The size of the volume that can be imaged is almost arbitrary, limited only by the penetration depth and the available scanning time. Here we present a method that rapidly accelerates the imaging operation over a given volume through acquiring a limited set of data via large angular reduction and compensating for the resulting ill-posedness through deeply learned priors. The proposed 3D reconstruction method “RAPID” relies initially on a subset of the object measured with the nominal number of required illumination angles and treats the reconstructions from the conventional two-step approach as ground truth. It is then trained to reproduce equal fidelity from much fewer angles. After training, it performs with similar fidelity on the hitherto unexamined portions of the object, previously not shown during training, with a limited set of acquisitions. In our experimental demonstration, the nominal number of angles was 349 and the reduced number of angles was 21, resulting in a
$$\times 140$$
×
140
aggregate speedup over a volume of
$$4.48\times 93.18\times 3.92\, \upmu \text {m}^3$$
4.48
×
93.18
×
3.92
μ
m
3
and with
$$(14\,\text {nm})^3$$
(
14
nm
)
3
feature size, i.e.
$$\sim 10^8$$
∼
10
8
voxels. RAPID’s key distinguishing feature over earlier attempts is the incorporation of atrous spatial pyramid pooling modules into the deep neural network framework in an anisotropic way. We found that adjusting the atrous rate improves reconstruction fidelity because it expands the convolutional kernels’ range to match the physics of multi-slice ptychography without significantly increasing the number of parameters.
Date issued
2023-04-03Department
Massachusetts Institute of Technology. Department of Mechanical Engineering; Massachusetts Institute of Technology. Department of Electrical Engineering and Computer Science; Singapore-MIT Alliance in Research and Technology (SMART)Publisher
Springer Nature Singapore
Citation
eLight. 2023 Apr 03;3(1):7
Version: Final published version