Stomach tissue classification using autofluorescence spectroscopy and machine learning
Author(s)
Lim, Soo Y.; Yoon, Hong M.; Kook, Myeong-Cherl; Jang, Jin I.; So, Peter T. C.; Kang, Jeon W.; Kim, Hyung M.; ... Show more Show less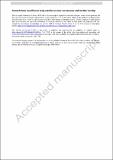
Download464_2023_10053_ReferencePDF.pdf (1.828Mb)
Open Access Policy
Open Access Policy
Creative Commons Attribution-Noncommercial-Share Alike
Terms of use
Metadata
Show full item recordAbstract
Abstract
Background and objectives
Determination of stomach tumor location and invasion depth requires delineation of gastric histological structure, which has hitherto been widely accomplished by histochemical staining. In recent years, alternative histochemical evaluation methods have been pursued to accelerate intraoperative diagnosis, often by bypassing the time-consuming step of dyeing. Owing to strong endogenous signals from coenzymes, metabolites, and proteins, autofluorescence spectroscopy is a favorable candidate technique to achieve this aim.
Materials and methods
We investigated stomach tissue slices and block specimens using a fast fluorescence imaging scanner. To obtain histological information from broad and structureless fluorescence spectra, we analyzed tens of thousands of spectra with multiple machine-learning algorithms and built a tissue classification model trained with dissected gastric tissues.
Results
A machine-learning-based spectro-histological model was built based on the autofluorescence spectra measured from stomach tissue samples with delineated and validated histological structures. The scores from a principal components analysis were employed as input features, and prediction accuracy was confirmed to be 92.0%, 90.1%, and 91.4% for mucosa, submucosa, and muscularis propria, respectively. We investigated the tissue samples in both sliced and block forms using a fast fluorescence imaging scanner.
Conclusion
We successfully demonstrated differentiation of multiple tissue layers of well-defined specimens with the guidance of a histologist. Our spectro-histology classification model is applicable to histological prediction for both tissue blocks and slices, even though only sliced samples were trained.
Date issued
2023-04-13Department
Massachusetts Institute of Technology. Department of Mechanical EngineeringPublisher
Springer US
Citation
Lim, Soo Y., Yoon, Hong M., Kook, Myeong-Cherl, Jang, Jin I., So, Peter T. C. et al. 2023. "Stomach tissue classification using autofluorescence spectroscopy and machine learning."
Version: Author's final manuscript