An inexact projected gradient method with rounding and lifting by nonlinear programming for solving rank-one semidefinite relaxation of polynomial optimization
Author(s)
Yang, Heng; Liang, Ling; Carlone, Luca; Toh, Kim-Chuan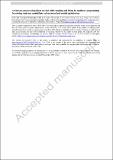
Download10107_2022_1912_ReferencePDF.pdf (13.09Mb)
Open Access Policy
Open Access Policy
Creative Commons Attribution-Noncommercial-Share Alike
Terms of use
Metadata
Show full item recordAbstract
Abstract
We consider solving high-order and tight semidefinite programming (SDP) relaxations of nonconvex polynomial optimization problems (POPs) that often admit degenerate rank-one optimal solutions. Instead of solving the SDP alone, we propose a new algorithmic framework that blends local search using the nonconvex POP into global descent using the convex SDP. In particular, we first design a globally convergent inexact projected gradient method (iPGM) for solving the SDP that serves as the backbone of our framework. We then accelerate iPGM by taking long, but safeguarded, rank-one steps generated by fast nonlinear programming algorithms. We prove that the new framework is still globally convergent for solving the SDP. To solve the iPGM subproblem of projecting a given point onto the feasible set of the SDP, we design a two-phase algorithm with phase one using a symmetric Gauss–Seidel based accelerated proximal gradient method (sGS-APG) to generate a good initial point, and phase two using a modified limited-memory BFGS (L-BFGS) method to obtain an accurate solution. We analyze the convergence for both phases and establish a novel global convergence result for the modified L-BFGS that does not require the objective function to be twice continuously differentiable. We conduct numerical experiments for solving second-order SDP relaxations arising from a diverse set of POPs. Our framework demonstrates state-of-the-art efficiency, scalability, and robustness in solving degenerate SDPs to high accuracy, even in the presence of millions of equality constraints.
Date issued
2022-11-29Department
Massachusetts Institute of Technology. Department of Aeronautics and AstronauticsPublisher
Springer Berlin Heidelberg
Citation
Yang, Heng, Liang, Ling, Carlone, Luca and Toh, Kim-Chuan. 2022. "An inexact projected gradient method with rounding and lifting by nonlinear programming for solving rank-one semidefinite relaxation of polynomial optimization."
Version: Author's final manuscript