Model-Based and Model-Free Point Prediction Algorithms for Locally Stationary Random Fields
Author(s)
Das, Srinjoy; Zhang, Yiwen; Politis, Dimitris N.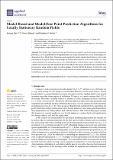
Downloadapplsci-13-08877-v2.pdf (1.657Mb)
Publisher with Creative Commons License
Publisher with Creative Commons License
Creative Commons Attribution
Terms of use
Metadata
Show full item recordAbstract
The Model-Free Prediction Principle has been successfully applied to general regression problems, as well as problems involving stationary and locally stationary time series. In this paper, we demonstrate how Model-Free Prediction can be applied to handle random fields that are only locally stationary such as pixel values over an image or satellite data observed on an ocean surface, i.e., they can be assumed to be stationary only across a limited part over their entire region of definition. We construct novel one-step-ahead Model-Based and Model-Free point predictors and compare their performance using synthetic data as well as images from the CIFAR-10 dataset. In the latter case, we demonstrate that our best Model-Free point prediction results outperform those obtained using Model-Based prediction.
Date issued
2023-08-01Department
Sloan School of ManagementPublisher
Multidisciplinary Digital Publishing Institute
Citation
Applied Sciences 13 (15): 8877 (2023)
Version: Final published version